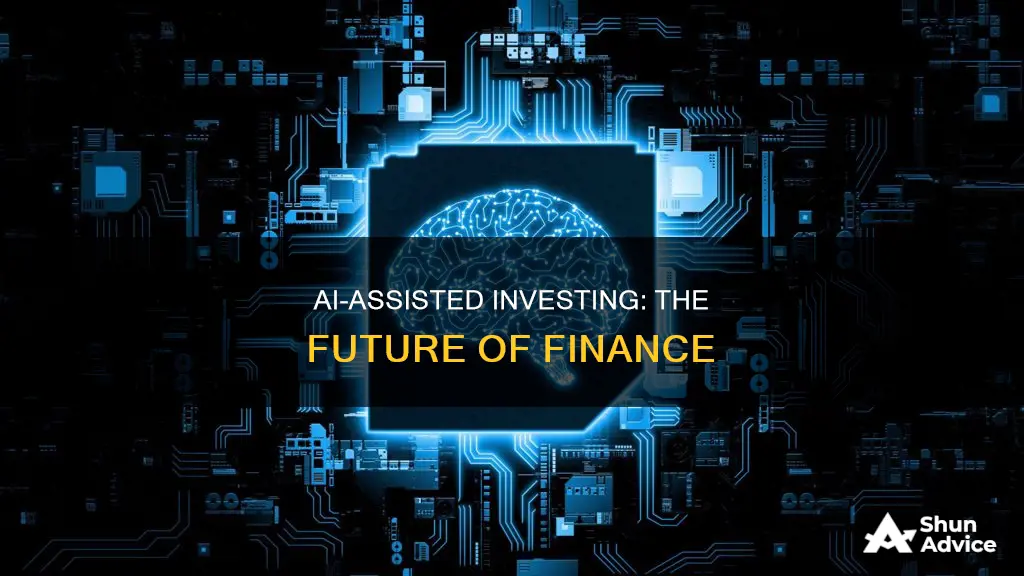
Artificial intelligence (AI) is no longer a futuristic concept. AI is increasingly being used in investing and finance, and investors are eager to capitalise on this rapidly growing industry.
AI can be used to help choose stocks, make predictions on market movement, optimise portfolios, manage risk, obtain personalised investment advice, and automatically build a customised portfolio that meets specific investor criteria like risk tolerances.
AI can also be used for algorithmic trading, sentiment analysis, portfolio optimisation, and risk management.
There are several ways to invest in AI, including buying stocks of public companies that develop AI software and manufacture the hardware that runs AI applications, or investing in exchange-traded funds (ETFs) that focus on AI-related investments.
Characteristics | Values |
---|---|
AI's use in investing | Algorithmic trading, sentiment analysis, portfolio optimization, personalized investment advice, etc. |
AI's impact on the economy | AI could raise global GDP by 14% by 2030. |
AI's impact on employment | AI has the potential to displace some jobs and create new ones, transforming the global labor force. |
AI's impact on wages and corporate profits | AI could boost wages and corporate profits. |
AI's impact on government budgets | AI could improve government budgets through taxes on higher incomes of workers and firms. |
AI's impact on globalization | AI could spur a new type of globalization in services. |
AI's impact on labor costs | AI could help manage labor costs by filling gaps and boosting output per worker. |
AI's impact on productivity | AI could drive faster productivity growth. |
AI's impact on investment | AI could lead to business investment climbing as benefits to businesses become more evident. |
AI's impact on data privacy | There are costs tied to regulatory uncertainty and data privacy issues. |
AI's impact on regulation | AI stocks face tougher regulation and legislation as agencies and lawmakers work on putting safety boundaries on the development and uses of AI. |
What You'll Learn
Algorithmic trading
How Algorithmic Trading Works
Algo-trading uses computer programs that follow a set of predefined instructions or algorithms to identify and execute trades. These instructions are typically based on factors such as timing, price, quantity, or mathematical models. For example, a trader might set up an algorithm to buy 50 shares of a stock when its 50-day moving average goes above the 200-day moving average and sell when it goes below. The algorithm will monitor the stock price and place the buy and sell orders automatically when the specified conditions are met.
Advantages of Algorithmic Trading
- Best Execution: Algo-trading often results in trades being executed at the best possible prices due to the speed and accuracy of computer programs.
- Low Latency: Trade orders are placed instantly and accurately, reducing the risk of significant price changes.
- Reduced Transaction Costs: Algo-trading can lead to lower trading fees.
- Simultaneous Checks: Multiple market conditions can be monitored and analysed simultaneously.
- No Human Error: The risk of manual errors or emotional factors influencing trading decisions is eliminated.
- Backtesting: Algo-trading strategies can be tested using historical and real-time data to evaluate their viability.
Disadvantages of Algorithmic Trading
- Latency: Reliance on fast execution speeds and low latency means that any delays in trade execution can result in missed opportunities or losses.
- Black Swan Events: Algo-trading relies on historical data and mathematical models, which may not account for unforeseen market disruptions.
- Dependence on Technology: Technical issues or failures can disrupt the trading process and result in losses.
- Market Impact: Large algorithmic trades can significantly impact market prices, affecting other traders.
- Regulation: Algo-trading is subject to complex regulatory requirements and oversight.
- High Capital Costs: The development and implementation of algo-trading systems can be costly, and ongoing fees for software and data feeds may be required.
- Limited Customization: Algo-trading systems follow predefined rules, which may limit traders' ability to customise their trades.
- Lack of Human Judgment: Algo-trading relies on mathematical models and historical data, which may not account for subjective or qualitative factors influencing market movements.
There are several common strategies used in algo-trading, including trend-following strategies, arbitrage opportunities, index fund rebalancing, mathematical model-based strategies, trading range or mean reversion strategies, and volume-weighted average price (VWAP) or time-weighted average price (TWAP) strategies.
Insurance: A Safe Investment Bet?
You may want to see also
Sentiment analysis
Artificial intelligence is increasingly being used in investing, with investors seeking to use its power to beat the market. AI can be used for sentiment analysis, which is a difficult task to perform manually due to the qualitative nature of sentiment data. AI programs can help traders assess market sentiment by collecting news articles, social media posts, and other online activity to measure market sentiment and predict movements.
For example, if AI detects a generally positive sentiment towards a particular stock or industry, investors may choose to buy stocks in that area. On the other hand, if AI identifies negative sentiment or emerging risks, investors may decide to sell their stocks or avoid investing in that sector. Thus, sentiment analysis can help investors make more strategic decisions and potentially improve their investment returns.
In addition to sentiment analysis, AI is also used in investing through algorithmic trading, portfolio optimization, and personalized investment advice. AI algorithms can analyze large datasets and execute trades at high speeds, taking advantage of market trends and patterns. AI can also assist in portfolio optimization by helping investors identify a portfolio that fits their specific needs, such as risk tolerance and time horizon.
Furthermore, AI-powered apps like Magnifi use generative AI and other tools to provide real-time, personalized investment advice to users. As AI continues to advance and become more accessible, it is likely that its use in investing will only grow, creating new opportunities for investors to leverage its capabilities.
Why Invest in Entertainment?
You may want to see also
Portfolio optimization
- Improved asset allocation: AI can assess various factors, including financial goals, risk tolerance, and market conditions, to determine the optimal mix of asset classes. It provides insights and recommendations to maintain a well-balanced portfolio aligned with the investor's objectives.
- Enhanced diversification: AI identifies correlations between different assets, helping investors spread their investments across various asset classes, industries, and sectors to reduce overall risk.
- Dynamic adjustments: AI continuously monitors the portfolio and market conditions, suggesting adjustments to maintain the desired asset allocation and enabling investors to adapt to changing market dynamics.
- Risk management: AI analyzes historical data, market trends, and investor profiles to assess and manage risk effectively. It can identify potential risks, such as market fluctuations, credit defaults, and liquidity issues, and provide proactive risk mitigation strategies.
- Performance optimization: AI optimizes the risk-return balance by employing advanced algorithms and predictive analytics. It aids in maximizing returns while minimizing risk, leading to improved overall portfolio performance.
Investing: Nice People Turn Nasty
You may want to see also
Risk management
Artificial intelligence (AI) and machine learning (ML) are increasingly being used in risk management within the financial services industry, particularly in banking. AI and ML tools, with their advanced prediction techniques and ability to utilise large volumes of data, are being leveraged to make quicker and more efficient credit, investment, and business-related decisions.
Superior Forecasting Accuracy
Traditional regression models often fail to capture non-linear relationships between the macro economy and company financials, especially during stressed scenarios. Machine learning offers improved forecasting accuracy due to its ability to capture nonlinear effects between scenario variables and risk factors.
Optimised Variable Selection Process
Feature/variable extraction processes can be time-consuming for risk models used for internal decision-making purposes. ML algorithms, when combined with big data analytics platforms, can process vast amounts of data and extract multiple variables. This enables the development of robust, data-driven risk models for stress testing.
Richer Data Segmentation
ML algorithms enable superior data segmentation and consider many attributes of segment data. By using unsupervised ML algorithms, it is possible to combine distance and density-based approaches for clustering, resulting in higher modelling accuracy and explanatory power.
Credit Risk Modelling
While banks traditionally use traditional credit risk models, ML models can be used to optimise parameters and improve the variable selection process in these models. AI-based decision tree techniques can result in easily traceable and logical decision rules, even with non-linear characteristics.
Fraud Detection
Credit card payment systems use workflow engines to monitor card transactions and assess the likelihood of fraud. The rich transaction history available for credit card portfolios enables banks to distinguish between specific features present in fraudulent and non-fraudulent transactions.
Trader Monitoring
Technologies such as natural language processing and text mining are used to monitor trader activity for rogue trading, insider trading, and market manipulation. By analysing email traffic, calendar data, check-in/check-out times, call times, and trading portfolio data, systems can predict the probability of trader misconduct, saving financial institutions millions in reputational and market risk.
Model Risk Management and Stress Testing
AI/ML-powered risk management solutions can be used for model risk management (back-testing and model validation) and stress testing, as required by global prudential regulators.
Operational Efficiency and Cost Reduction
AI/ML solutions can generate large amounts of timely and accurate data, allowing financial institutions to build competence around customer intelligence and lower potential losses. Additionally, these technologies can enhance the overall customer experience by streamlining operations and automating processes.
Why Early College Programs Are Worth the Investment
You may want to see also
Personalised investment advice
Artificial intelligence (AI) is increasingly being used to provide personalised investment advice. AI tools can help financial advisors with a range of tasks, including client service, data analysis, portfolio optimisation, risk assessment, and trend prediction.
AI can be used to identify trends and patterns in historical data that humans may not be able to see. It can also monitor keywords, social media and online content to spot trending topics and provide more up-to-date investment advice. AI can also be used to create infographics and blog content to communicate this advice.
AI tools can also be used to improve risk management for clients. With this technology, financial advisors can help their clients align their portfolios with their risk tolerance levels and reduce losses. AI can also play a role in portfolio optimisation, helping to balance diversification, risk, and factors like income and growth.
AI-powered apps, such as Magnifi, use ChatGPT and other AI tools to provide real-time, personalised investment advice. According to Andrew Lo, a professor of finance at MIT Sloan, AI has the potential to provide sound, personalised financial advice, but only with the addition of supplemental modules that incorporate finance-specific knowledge. Lo also notes that AI tools can be used to personalise the tone of the advice given, depending on the client's level of optimism or pessimism.
Mortgage Applicants: Lying About Investment Properties
You may want to see also
Frequently asked questions
Artificial intelligence (AI) is the use of machines to simulate human intelligence. AI is accomplished by computers and software, and uses data analysis and rules-based algorithms.
AI is used in investing in several ways, including algorithmic trading, sentiment analysis, portfolio optimization, and personalized investment advice. AI can help identify trends, improve diversification, and make investment decisions.
AI can process and analyse large datasets much faster than humans, giving an advantage in high-frequency trading. It can also remove human biases from investment decisions and improve diversification.
AI applications for financial markets are only as good as the quality of the application and the user's ability. There are also regulatory and ethical considerations, as well as the potential for high costs and labour displacement.