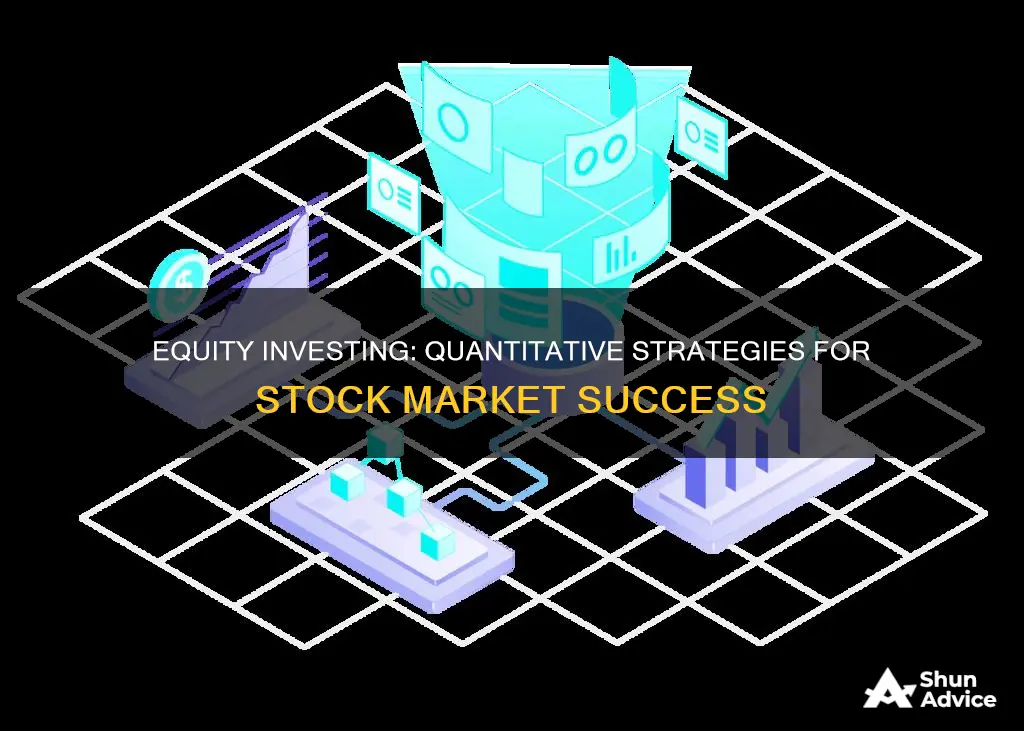
Quantitative equity investing is a data-driven approach to investment decision-making that uses advanced mathematical modelling, computer systems, and data analysis to calculate the optimal probability of executing a profitable trade. It involves creating and evaluating trading strategies using rigorous rule sets to decide when and what to trade. This method, also known as systematic investing, aims to remove the emotional element from investing by using quantitative techniques and tools such as machine learning, mathematical modelling, factor investing, and alternative data analysis.
Characteristics | Values |
---|---|
Definition | Quantitative equity investing is an investment approach that uses advanced mathematical modelling, computer systems and data analysis to calculate the optimal probability of executing a profitable trade. |
Other names | Systematic investing |
History | It was historically the domain of sophisticated hedge funds, but as computational power and data storage have become more accessible, traditional institutional investors and fundamental funds have begun to borrow quantitative techniques and tools. |
Examples | High-frequency trading, algorithmic trading, and statistical arbitrage |
Books | Principles of Quantitative Equity Investing: A Complete Guide to Creating, Evaluating, and Implementing Trading Strategies; Quantitative Equity Investing: Techniques and Strategies; Quantitative Equity Portfolio Management, Second Edition: An Active Approach to Portfolio Construction and Management; Advanced Portfolio Management: A Quant's Guide for Fundamental Investors |
Authors | Sugata Ray; Frank J. Fabozzi; Sergio M. Focardi; Petter N. Kolm; Ludwig B. Chincarini; Giuseppe A. Paleologo |
What You'll Learn
Mathematical modelling and data analysis
Quantitative equity investing involves using mathematical modelling and data analysis to identify profitable trading opportunities.
The process typically begins with building a mathematical model of the proposed trading strategy. This model is then backtested using historical market data to evaluate its effectiveness. This backtesting process is crucial as it allows investors to assess the performance of their strategy over different historical time periods and market conditions. However, it is important to be cautious about overfitting the model to the specific time period or market conditions being tested.
Mathematical modelling in quantitative equity investing involves using advanced mathematical techniques and computer systems to analyse market data and identify patterns. This includes the use of financial econometrics, regression analysis, random matrix theory, dynamic time series models, and vector autoregressive models. These models help investors understand the relationships between different financial variables and make informed decisions about their investments.
Data analysis plays a crucial role in quantitative equity investing. Investors analyse large datasets to identify market trends, anomalies, and predictive signals. This includes analysing traditional financial metrics as well as alternative data sources such as social media sentiment and news articles. By incorporating diverse data sources, investors can gain a competitive edge and make more informed decisions.
In addition to backtesting, stress testing is also employed to assess the resilience of the models under extreme market scenarios. This helps investors identify potential risks and ensure that their strategies are robust and adaptable to different market conditions.
Overall, mathematical modelling and data analysis are essential tools in quantitative equity investing. They enable investors to develop data-driven strategies, manage risk, and identify profitable trading opportunities. By utilising these techniques, investors can make more informed and rational decisions, ultimately aiming to maximise their profits and control their risk exposure.
Savings or Investment Plans: Where Should Your Money Go?
You may want to see also
High-frequency and algorithmic trading
High-Frequency Trading (HFT)
High-frequency trading is a subset of algorithmic trading, characterised by high speeds, high turnover rates, and high order-to-trade ratios. HFT algorithms capitalise on minuscule price discrepancies, executing trades within milliseconds or even microseconds. This ultra-fast trading seeks to exploit fleeting arbitrage opportunities and profit from infinitesimal price movements.
HFT algorithms act as middlemen, dynamically controlling the timing and placement of orders. They analyse real-time data, detect trading signals, identify price levels, and place orders accordingly. A key strategy is to sense pending large-size orders by sending multiple small-sized orders and analysing the patterns and execution times.
HFT has been controversial, with critics arguing that it contributes to market volatility and fragility. The "Flash Crash" of May 6, 2010, was partially attributed to HFT activities, where high-frequency liquidity providers rapidly withdrew from the market. However, supporters of HFT argue that it improves market efficiency, increases liquidity, and assists in price discovery and formation.
Algorithmic Trading (AT)
Algorithmic trading, or algo-trading, uses computer programs to execute trades based on predefined instructions and mathematical models. It aims to remove human emotions from trading, increase efficiency, and capture profitable opportunities at speeds impossible for human traders.
Algo-trading is based on factors such as timing, price, quantity, and mathematical models. Common strategies include trend-following, arbitrage, and index fund rebalancing. It also employs volume-weighted and time-weighted average price strategies to manage large orders and minimise market impact.
The advantages of algo-trading include best execution, low latency, reduced transaction costs, and the elimination of human error. However, there are drawbacks, such as the reliance on technology, high capital costs, limited customisation, and the potential for increased market volatility.
In conclusion, high-frequency and algorithmic trading have transformed the investment landscape, offering new opportunities and challenges. While they provide benefits such as efficiency and liquidity, they also raise concerns about market stability and fairness. As computational power continues to advance, these trading methods will likely become even more prevalent and sophisticated.
Understanding Diverse Investment Portfolio Types and Their Benefits
You may want to see also
Factor investing
There are several commonly used factors in factor investing:
- Value factor: This focuses on stocks that are undervalued using metrics like price-to-earnings or price-to-book ratios.
- Momentum factor: This involves targeting stocks that have demonstrated strong price performance over a specific time frame.
- Size factor: This focuses on smaller companies that tend to have higher growth potential.
- Quality factor: This looks at companies with strong fundamentals, such as a high return on equity, low debt, and stable earnings.
- Volatility factor: This focuses on low-volatility stocks that are less susceptible to market swings.
- Yield factor: This involves targeting high-dividend stocks.
Rebalancing Your Investment Portfolio: How Often is Necessary?
You may want to see also
Risk-parity approach
Quantitative investment strategies use mathematical models and algorithms to identify investment opportunities. One such strategy is the risk-parity approach, which seeks to allocate capital based on the risk of each asset in a portfolio rather than on expected returns. This strategy is a modification of the modern portfolio theory (MPT) approach, which seeks to diversify an investment portfolio among specified assets to optimise returns while adhering to market risk parameters.
The risk-parity approach aims to balance risk across various asset classes like equities, bonds, commodities, and alternative investments. It does so by using leverage to increase investments in lower-risk assets, such as bonds, to offset the risk contributions from each asset class. This strategy is often used by hedge funds and sophisticated investors and requires a complex quantitative methodology.
The goal of the risk-parity approach is to earn the optimal level of return at the targeted risk level. It allows for the use of leverage, alternative diversification, and short selling in portfolios and funds. Portfolio managers can use any mix of assets they choose but instead of generating allocations to different asset classes, they use the optimal risk target level as their basis for investing. This is often achieved by using leverage to weight risk equally among different asset classes.
For example, a portfolio with a 100% allocation to equities has a risk of 15%. By using moderate leverage of around 2.1 times the amount of capital, a portfolio with 35% allocated to equities and 65% to bonds can achieve the same expected return but with an annualised risk of only 12.7%. This is a 15% reduction in risk.
The risk-parity approach also uses the security market line (SML), which is a graphical representation of the relationship between the risk and return of an asset. The SML is used in the capital asset pricing model (CAPM), with the slope of the line determined by the beta of the market. The greater the possibility for the return of an asset, the higher the risk associated with that asset.
However, the risk-parity approach is not without its challenges. It is sensitive to estimating risk parameters and correlations among assets, which can change over time. The use of leverage can also amplify losses during adverse market conditions. Despite these complexities, the risk-parity approach is favoured as it tends to deliver more stable and diversified returns.
Understanding India VIX: A Guide to Investing in Volatility
You may want to see also
Machine learning and artificial intelligence
AI and machine learning are being leveraged to identify patterns and trends in market behaviour, optimise portfolio allocation, and enhance risk management. The power of these technologies lies in their ability to process vast amounts of data and identify complex patterns that traditional methods might overlook. This enables investment managers to make more informed decisions and potentially improve their investment returns.
The application of AI in quantitative investing involves utilising machine learning algorithms to analyse granular data and develop models that better reflect the complexities of the real world. This contrasts with traditional quantitative analysis, which often simplifies the investment environment by targeting specific factors that can drive returns. By incorporating more data points and variables, AI-based models can provide a more nuanced understanding of market dynamics and investment opportunities.
However, there are challenges to the widespread adoption of machine learning and AI in this field. One challenge is the low signal-to-noise ratio in financial data, where any given metric may not have a significant impact on a security's performance due to various influencing factors. Additionally, the lack of extensive historical financial data and the evolving nature of financial markets pose obstacles to the training and accuracy of machine learning models.
Despite these challenges, the future of quantitative equity investing is expected to be shaped by the continued integration of AI and machine learning. The potential benefits of enhanced risk management, improved insights, and more efficient trade execution make this a compelling area of focus for investment professionals. The combination of human intelligence and AI-driven analytics is likely to be a key driver of success in the investment landscape.
Unlocking Private Equity's Integrated Investment Strategy Secrets
You may want to see also
Frequently asked questions
Quantitative equity investing is an investment approach that uses advanced mathematical modelling, computer systems, and data analysis to calculate the optimal probability of executing a profitable trade.
Examples include high-frequency trading, algorithmic trading, and statistical arbitrage.
Quantitative equity investing offers a data-driven approach to investment decision-making, reducing the influence of emotions and biases. It also provides transparency in the investment process and allows for backtesting of strategies before implementation.
Some potential risks and challenges include model risk, overfitting, market changes, computational complexity, market impact, liquidity issues, and regulatory and ethical concerns.