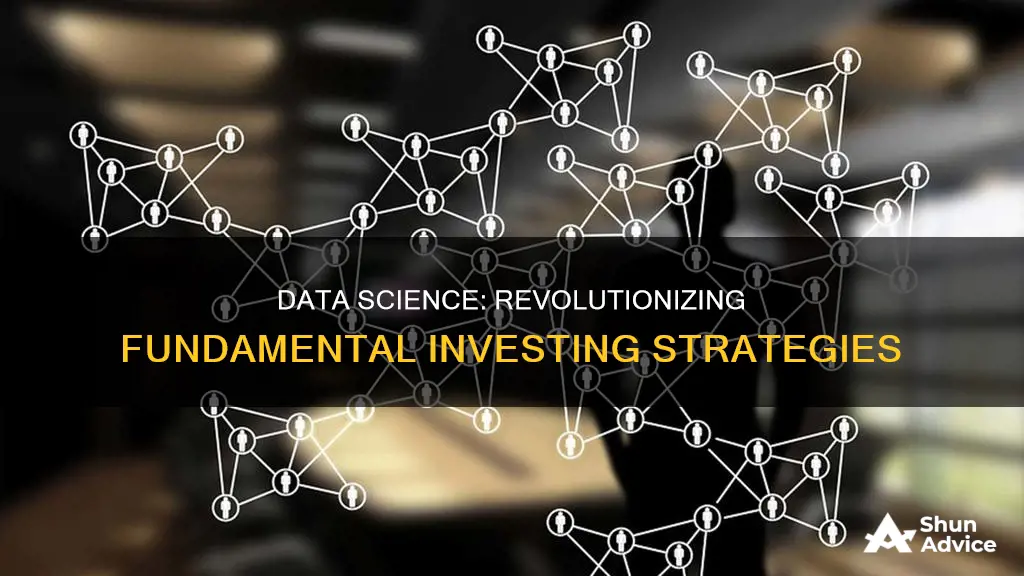
Data science is an increasingly popular tool for investors, with the potential to transform the investment industry. By employing data analytics, investors can make more informed decisions, optimise investment strategies, and identify opportunities and risks. This is particularly useful in the unpredictable world of finance and investment, where data science can be used to forecast future market trends and consumer behaviour.
Data science tools can be used to collect and analyse financial data, such as bank statements, stock prices, and interest rates, and to handle this sensitive information ethically. This data can then be used to create models and predictions for future investments and financial decision-making. For example, data science can be used to identify undervalued companies by analysing a company's financial statements, revenue growth, profitability, and broader economic indicators.
Additionally, data science can be used to develop algorithmic trading strategies, which execute trading orders based on programmed instructions and historical data. This allows for more precise and timely trades, minimising errors. Data science can also be used for automated risk management and fraud detection, enhancing the security and transparency of financial transactions.
Overall, data science has the potential to bring significant advantages to the field of investing, providing investors with valuable insights and predictive capabilities.
Characteristics | Values |
---|---|
Purpose | To identify opportunities, mitigate risks, track performance, and make decisions backed by hard evidence |
Data Types | Price, volume, and news |
Benefits of Real-time Data | Keeping traders ahead with the latest market updates, crucial for making swift trades and adjustments based on current market dynamics |
Benefits of Historical Data | Offers insights into past market performance, helping identify trends and test trading strategies against historical outcomes for informed investment decisions |
Risk Management | Real-time data allows for immediate risk mitigation, while historical data facilitates the assessment of strategy viability over different market conditions |
Portfolio Optimization | Historical insights guide long-term investment decisions and portfolio structuring, while real-time updates enable quick reactions to market opportunities for short-term gains |
Regulatory Compliance | Advanced analytics help firms identify and rectify compliance issues promptly, ensuring adherence to regulations and minimizing the risk of violations |
Data Science Applications | Real-time market insights, algorithmic trading, automated risk management and fraud detection, optimization of pricing and revenue, sentiment analysis and news summarization |
Data Science Tools | Natural Language Processing (NLP), machine learning, and programming languages such as R or Python |
What You'll Learn
- Data science can be used to identify opportunities and risks
- It can help to track performance and make evidence-based decisions
- Data science can be used to make predictions about future market trends
- It can be used to perform risk assessments and credit scores
- Data science can be used to inform algorithmic trading
Data science can be used to identify opportunities and risks
Market Dynamics and Trends
Data analytics plays a pivotal role in understanding market dynamics and extracting market trends. By analysing extensive datasets, investors can identify patterns, market movements, and predictive indicators. This allows them to make informed decisions and optimise their investment strategies. For example, by studying price data, volume data, and news data, investors can discern market trends, assess market interest, and pinpoint strategic entry and exit points.
Risk Management and Compliance
Data science facilitates effective risk management by providing real-time data and advanced analytics tools. Real-time data allows investors to make immediate adjustments to their portfolio exposure and quickly react to market changes. Advanced analytics tools help in identifying and rectifying compliance issues, ensuring adherence to regulations and minimising the risk of violations. Additionally, data science models can evaluate historical data to predict potential risks and suggest portfolio adjustments.
Enhanced Decision-Making
Data-driven investing provides investors with specific financial insights, enabling them to make decisions backed by hard evidence. By analysing different data sources, investors can identify new opportunities, perform precise risk assessments, and discover market trends. Data science also enhances the accuracy of models and predictions, minimising human error and cognitive biases. This allows investors to make more informed choices and potentially improve their long-term investment outcomes.
Fraud Detection and Security
Data science, particularly machine learning algorithms, excels at identifying irregular patterns indicative of fraud. Financial institutions can leverage these capabilities to safeguard their assets and proactively defend their interests. Additionally, blockchain technology, often associated with data science, fortifies security and transparency in financial transactions, further reducing the risk of fraud and enhancing the integrity of the financial system.
Optimisation of Pricing and Revenue
Data science algorithms can fine-tune pricing strategies and maximise revenue potentials. By analysing investor behaviours and preferences, data-driven approaches can lead to customised investment services, improving investor satisfaction and retention. Data-informed pricing decisions have been shown to significantly boost profitability and enhance revenue opportunities.
T. Rowe Price Employee Investment Requirements: What You Need to Know
You may want to see also
It can help to track performance and make evidence-based decisions
Data science can be used to track performance and make evidence-based decisions in fundamental investing by employing advanced analytics tools and methodologies. It enables investors to monitor financial performance automatically and make real-time adjustments to their portfolios. For instance, data science algorithms can be used to track and analyse key financial data such as stock prices, interest rates, and bank statements. This allows investors to identify patterns, market trends, and potential risks, facilitating more informed decision-making.
Additionally, data science enhances risk management capabilities. By leveraging historical data, investors can assess the viability of their investment strategies over different market conditions and make data-driven adjustments to optimise their portfolios. Data science also aids in regulatory compliance by helping firms identify and address compliance issues promptly, thus minimising the risk of violations.
Furthermore, data science enables investors to make evidence-based decisions by providing predictive insights. Through the analysis of extensive datasets, investors can forecast market movements and identify investment opportunities. Data science algorithms can process vast amounts of data, including news articles, social media content, and financial reports, to assess market sentiment and make strategic investment choices.
Data science also plays a crucial role in algorithmic trading. By utilising programmed instructions based on financial data, trading orders can be executed automatically, eliminating the need for manual monitoring and order placement. This further emphasises the role of data science in tracking performance and facilitating evidence-based decisions in fundamental investing.
Overall, data science empowers investors with the ability to track financial performance, identify trends and risks, and make data-driven adjustments to their portfolios. By leveraging advanced analytics and predictive insights, investors can enhance their decision-making capabilities and optimise their investment strategies.
Where to Park Your Excess Cash?
You may want to see also
Data science can be used to make predictions about future market trends
Data science professionals use this data to create models and predictions for safer investments and financial decision-making. For example, data science professionals working with the stock market can create models for trading stocks or predicting which stocks will be the most successful in the future based on past performance. Data science can also be used to perform risk assessments on investments and to determine whether a bank should loan money to a particular individual or institution.
Data science tools and programs are particularly useful for making predictions or forecasts about future activity based on past trends. By learning programming languages such as R or Python, data science professionals can create predictive models based on past occurrences.
In addition to predicting future market trends, data science can also be used for algorithmic trading. Algorithmic trading uses programmed instructions based on financial data to execute trading orders. For example, a trader might programme their computer to buy 50 shares of a stock when its 5-day moving average goes above the 20-day moving average, and to sell the shares when the 5-day moving average goes below the 20-day moving average.
Cash Investments: Recording Entries and Their Impact
You may want to see also
It can be used to perform risk assessments and credit scores
Data science can be used to perform risk assessments and credit scores, helping to create more accurate models and predictions of future market trends and consumer behaviour. For example, data science can be used to predict future market trends and consumer behaviour, which can inform investment strategies and risk management. This can involve analysing historical financial data, such as bank statements, stock prices, and interest rates, to identify patterns and make informed decisions about investments.
Data science can also be used to perform credit scores, which are used to assess the creditworthiness of individuals or businesses. This involves analysing financial data such as credit history, income, and existing debt to determine the likelihood of an individual or business repaying their debts. This information can then be used by lenders to make informed decisions about whether to extend credit and on what terms.
In addition, data science can be used to develop algorithmic trading systems, which use programmed instructions based on financial data to automatically buy or sell assets. These systems can take into account various factors, such as stock price movements and market trends, to make trading decisions in real-time.
Data science also enhances risk management by enabling the development of sophisticated models that can evaluate historical data to predict potential risks and suggest portfolio adjustments. This helps financial institutions to proactively defend their interests and minimise losses.
Furthermore, data science enables the use of machine learning algorithms to identify irregular patterns indicative of fraud, enhancing the security and transparency of financial transactions. By analysing large datasets, these algorithms can detect fraudulent activities and protect the assets of financial institutions and their clients.
Millionaires' Secrets: Where They Invest Their Money
You may want to see also
Data science can be used to inform algorithmic trading
Historical data can be used to identify trends and test trading strategies against historical outcomes. Real-time data, on the other hand, is crucial for making swift trades and adjustments based on current market dynamics. For example, a data analytics dashboard could alert a portfolio manager to a possible labour strike several hours before the news hits the market, allowing them to assess the impact of the event on the company's fundamental value and make a portfolio decision.
Data science can also be used to create predictive models based on past occurrences. For instance, data science professionals can create models for trading stocks or predicting which stocks will be the most successful in the future based on how similar investments have performed in the past.
Additionally, data science can be used to perform risk assessments on investments and determine whether a bank should loan money to a particular individual or institution based on data from their financial history. This can be done through sophisticated risk management models that evaluate historical data to predict potential risks and suggest portfolio adjustments.
Finally, data science can be used to detect and prevent fraud. Machine learning algorithms can identify irregular patterns indicative of fraud, enabling financial institutions to safeguard their assets effectively.
Building an Investment Model: Python Strategies and Techniques
You may want to see also
Frequently asked questions
Fundamental investing is a method of determining a stock's intrinsic value by examining a company's financial statements and broader economic indicators. This analysis gives an investor the true worth of an investment based on a company's financial health, the market, and economic conditions.
Data science brings predictability to the unpredictable world of finance and investment. It helps investors identify opportunities, mitigate risks, track performance, and make decisions backed by hard evidence.
The three crucial types of data used are price data, volume data, and news data. Price data includes open, high, low, and close prices. Volume data indicates market interest and activity. News data is compiled from press releases, financial news, and social media, providing insights into market sentiment.
Historical data is useful for testing trading strategies and identifying trends over time. Real-time data, on the other hand, keeps investors ahead of the game with the latest market updates, allowing for swift trades and adjustments.
Data science facilitates risk management by evaluating historical data to predict potential risks and suggest portfolio adjustments. Machine learning algorithms can also identify irregular patterns indicative of fraud, helping financial institutions safeguard their assets.